AI for Medicine
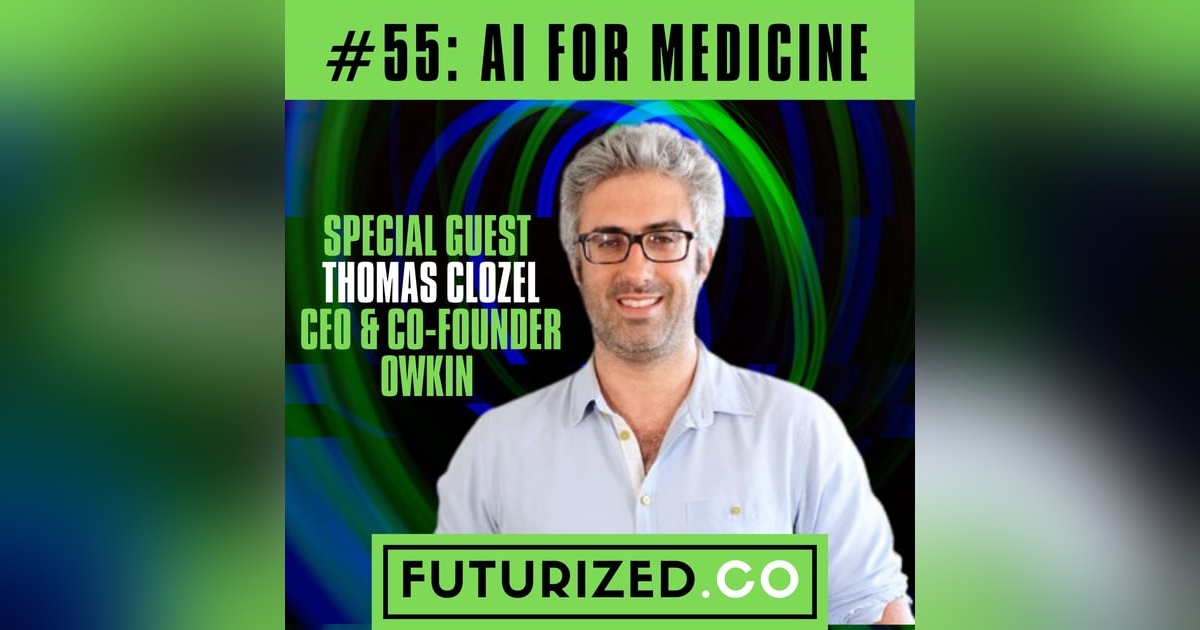
Thomas Clozel, CEO and co-founder of Owkin, the federated data network startup boosting AI for medicine, interviewed by futurist Trond Arne Undheim.
In this conversation, they talk about integrating AI and system biology to enable breakthrough medical m...
Thomas Clozel, CEO and co-founder of Owkin, the federated data network startup boosting AI for medicine, interviewed by futurist Trond Arne Undheim.
In this conversation, they talk about integrating AI and system biology to enable breakthrough medical moments. They explore how machine learning can be used to augment medical and biological research. In Owkin’s federated learning approach, the data stays put with the owners, but the learning models travel. Theydiscuss the data heterogeneity in healthcare, the need for interoperability, and we touch on hype versus reality.
The takeaway is that AI for medicine is here to stay, it has come far, but has bumped into some fundamental obstacles—interoperability, explainability, privacy, and transparency—that need to be resolved before reaching its full potential. That’s even more important than evolving the base technology to become more efficient. This is why federated learning is such a crucial experiment. Can it work? Will it satisfy everyone? Time will show. AI is not monolithic and neither is medicine. There seems to be many contenders for glory, and there are many puzzles to solve. They won’t all go away this decade.
After listening to the episode, check out Owkin as well as Thomas Clozel's online presence:
- Owkin (@OWKINscience) https://owkin.com/
- Thomas Clozel (@TClozel) https://www.linkedin.com/in/thomas-clozel-m-d-408a9321/
The show is hosted by Podbean and can be found at Futurized.co. Additional context about the show, the topics, and our guests, including show notes and a full list of podcast players that syndicate the show can be found at https://trondundheim.com/podcast/. Music: Electricity by Ian Post from the album Magnetism.
For more about the host, including media coverage, books and more, see Trond Arne Undheim's personal website (https://trondundheim.com/) as well as the Yegii Insights blog (https://yegii.wpcomstaging.com/). Undheim has published two books this year, Pandemic Aftermath and Disruption Games. To advertise or become a guest on the show, contact the podcast host here. If you like the show, please subscribe and consider rating it five stars.
AI for Medicine_mixdown
Trond Undheim, Host: [00:00:00] Futurize goes beneath the trends to track the underlying forces of disruption in technology, policy, business models, social dynamics, and the environment. I'm your host through Ana in Haim, futurist and author in episode 55 of the podcast, the topic is AI for medicine. Our guest is Thomas Clozel, CEO, and founder of Owkin.
[00:00:25] The federated. Data networks, startup boosting AI for medicine in this conversation, we talk about integrating AI and systems biology to enable breakthrough medical moments. We explore how machine learning can be used to improve medical and biological research in Ocwens federated learning approach. The data stays with the owners, but the learning models travel we discussed.
[00:00:55] The data heterogeneity in healthcare, the need for interoperability. And we touched on hype versus reality. Thomas, how are you
[00:01:04] Thomas Clozel: [00:01:04] doing today? I'm great, Trond. So nice to be with you and yes, it's been a
[00:01:10] Trond Undheim, Host: [00:01:10] while. And what are you doing in Switzerland? What's going on?
[00:01:14] Thomas Clozel: [00:01:14] I know it's surprising. I surprised myself. We literally like were banned from the U S in March and we left the U S thinking like we're going to be in France.
[00:01:23] They in Brittany, they, the words are, and we realized we wouldn't be able to go back. So we had to choose anywhere. International is a good research period. And here we have Geneva and it's great that it's far,
[00:01:37] Trond Undheim, Host: [00:01:37] wow. COVID has really thrown a wrench into a lot of stuff including startup collaboration and people's lifestyles and and everything.
[00:01:45]Let's get into all of it. I wanted to kick it off though. Thomas, we met. At the founders forum, I believe. You're, heavily steeped in the founder environment for a while. So that's how I know you, but I know from your background that you have a past, as a clinical research professor in Paris, in hematology. So I wanted to bring that up and because I think it's probably relevant are a lot of people starting at AI startups these days with something to do with medical, but not all of them, have that much of a true medical research background. And then you've also been in the U S in that or Cornell Weill Cornell medical college and in New York a little bit.
[00:02:24]Other than that, I don't know too much about your background other than, you founded Okin right with Jill Wayne rib. So I wanted to ask you just as a kickoff here, what would you say in, in in your background has meant the most to you in your professional career and what has led you to where you are right now?
[00:02:43] Thomas Clozel: [00:02:43] Yeah. So the question, Sean, and so I'm an oncologist by training and I really wanted to learn how to treat patients and and trying to treat patients is a very complex problem that is totally unsolved today, especially in cancer, who would think that we would be here in cancer therapy. Of course there is a lot of progress.
[00:03:00] Is car T cells. Immunotherapy, but we still don't get it. We don't understand the biology. We don't understand why some people response to anti PD, one therapy, although they're, PD-L1 negative. We are keeping on coding. Some kids are by a negative terms, such as non-small cell lung cancer. We know it's non-small cell, but we don't know what it is.
[00:03:21] Or triple negative breast cancer. Okay. It's triple negative, but what is it? And we still have this really lack of comprehension and therefore what I studied to be a clinician, I was like I don't want to treat my patient as a patient on the, I want to understand how many clones is there. Isn't this cancer.
[00:03:35] I want to map the, can the clones understand the probability of relapse for Avery clone? And I really wanted to understand how to map out the virginity of the tumors and we understand her, how we could make, like more director changed the way we should patients. And I started to be interested in different technologies.
[00:03:53] And machine learning was one of the one that was the closest from the nature, because you can actually have nonlinear models that fits better to what's real. And I started to be very interested in this field.
[00:04:06] Trond Undheim, Host: [00:04:06] Thomas, I'm fascinated by this because, I have looked at this topic from various angles.
[00:04:11] And one of the things that strikes me with healthcare is that because it is so extremely specialized at times. To bring these two and it's not even just two I'm. Sure. But even just, if you take computer science and you add medical science, and then you actually add clinical medicine or the daily practice of actually being a doctor in a primary care situation and you meld and mold those two together, the initial matchup isn't very obvious at all.
[00:04:42] Is it? I don't know. I Both in terms of combining the knowledge, but also they are so different.
[00:04:50] Thomas Clozel: [00:04:50] Yeah, but I think every, I think like first you have to think about it. Future of physicians, right? What is a physician tomorrow? I see a physician as a meshing teacher, he's going to teach machines.
[00:04:59] He's teaching students, of course, teaching other people. He's also going to teach AI because AI is going to be part of his daily life. He's doing it when he's going on Facebook without knowing it. But he has to know how he's going to teach robots and machines to help him out in his practice. Okay.
[00:05:13] And really understanding that. I, and I think if you're a regular just today and you're not interested in AI, you're not interested in what's going to replace you. So it doesn't make sense. And I think like everyone should do that in a single show. Everyone should, could to be able to have some access to more knowledge by themselves.
[00:05:28]So Thomas,
[00:05:28] Trond Undheim, Host: [00:05:28] I love that you start from that side because one of the things that I really like about you. I know I admire so much is you're very humble. And to start with talking about the, kind of the weaknesses in your own original profession, and start from that point, I think is a very powerful message because a lot of people would start from the other angle.
[00:05:47] They would say AI is so advanced and these doctors have no idea, but they're, obviously it must be the other side of the coin, which is if you are a software programmer today. The amount of medical knowledge that you would really have a need to operate and be useful in an AI for medicine context.
[00:06:07] It wouldn't that also be very demanding.
[00:06:10] Thomas Clozel: [00:06:10] No. Yeah. I think firstly, everyone could have, this is humble when you tried to treat cancer patients, you have to be. And yeah, I think the it's. I think like the way to take the medical approach to AI versus the AI approach or the software engineering approach to medicine is complimentary.
[00:06:22] I think the way we operate as physicians is way more unsupervised. We know that we don't know. Which is a Socrates approach type of things. And so as far we actually want to find a few questions. I can imagine the answer by AI. AI is mostly trying to fit some models everywhere and trying to find things even that black books.
[00:06:38]And and I think it's we are there more like the way we think as physicians is we need to create knowledge here and there, and let's try to fill this gap. But I think today what's the most important thing in the field of AI is this connection between cubing, your needles and medical researchers.
[00:06:52] And a scientist and they need to work together. If you appear that a science company working with software engineers, and you don't have a medical background, you're going to build some things that are going to be black books, not useful, and you're not going to be able to actually have the usage of it.
[00:07:05] And I think understanding the performance is as important as adjusting the usage of this algorithm. And you can, you're going to miss this part. And the second thing is you can also miss what's the breakthrough within the models. Every models. If it's works, has some breakthroughs and features can be new targets of drugs and you really need to be able to understand what it means on the patient side and patient level.
[00:07:24] And I think this is yeah, the interactions between the two is very important and AI has to be interpretable for the physicians to actually understand how it works. But physicians also need to understand how they can teach machines to be better and how they can monitor. The performances of this models.
[00:07:39] This is very important as well in the acceleration phase of algorithm. If you don't know how to monitor all the performances and the benchmark, it's the reality. You can be a little bit like under the hype and a lot of things today in AI, he's hyped. I'm hot. I
[00:07:54] Trond Undheim, Host: [00:07:54] tell him this. I wanted to ask you about these two things.
[00:07:56]Before we, and we're going to talk about, precisely your approach, your chosen approach as well. But I wanted to do two things. First one, if you could map the AI for medicine space for us, put it in a little bit into categories of what we're doing, because that's number one. And then number two, we'll get to the hype versus reality.
[00:08:14] And then, I so let's start with mapping the AI space. What are the different things that even startups or practitioners or, how do you. Box this field,
[00:08:26] Thomas Clozel: [00:08:26] I'm sure you have a different answer and I'd have to hear it too, because you expressed the DMAT will give you a lot of feedback too.
[00:08:32] And so my feeling is like, so I always think AI as first, there's really two sides of it and I books it in two parts. Acceleration and orientation. It's really hope you got to think about it, right? The first is I really like how you actually accelerate processes and diagnosis. You go faster, you can do like a thousand CT scan reading per day.
[00:08:51] And the other one is augmentation. How do you get super powers? How can you predict from a CT scan of survival? How you can predict a response to treatments, how you can discover within the model. New genes that can be targets of innovative drugs. So I think the first way to really think about it is thinking about it.
[00:09:09] Do you want, are you in the augmentation and accelerate or acceleration phase? And the both are very different because of course I want attention is better. It's more exciting for doctors. It doesn't threaten the roles as a radiologist, for example. And so it gives them super powers to the new thing. So they really like it.
[00:09:25] But acceleration of course is also very important to on the daily workflow to make things more efficient. The second way to really think about AI. I think first, when you knew where you are in these two broads, What is the data access and the data preparation part and what is the data analytics side?
[00:09:39]And I think both are extremely important in the eye for me. The first is the most important one is how you access high quality data sets, especially in medical fields. There is a lot of regulation, GDPR being the most stringent in Europe. You also have HIPAA. And the second part is the analytics and the algorithm.
[00:09:55] And I think that how you actually find a really good technique to a way to access the best datasets to train and then the right algorithm to turn some things that works and how your model can be generalizable. This is the last part is. You need to think about the performances, your crazy, but you also need to think about rubbish nets and Huggies and generalize, the model.
[00:10:13] It's big problem for AI. Anyone can do it on the AI model on shelf. It works for his data, and then he never works on people's data. And then they use age. How you integrate this in the workflow is very important too. So I think these three levels of perfection, when I think about AI,
[00:10:29] Trond Undheim, Host: [00:10:29] That's fun. That's fascinating.
[00:10:31] Let's talk a little bit more about w data access, just because I know there's more coming here because you have this concept of federated learning before we get to Okin specifically, just explain to me what are the specific data sets that one typically thinks of when it comes to AI for medicine?
[00:10:49] Cause I know in the field of biomedicine, There's an enormous amount of databases and historically they weren't even cooperating. So they were competitors. Some of them are private. Some of them are locked down by law. And then there's an awful lot of databases that don't really have what you.
[00:11:07] As a computer scientist traditionally would think of as big data, or there are a lot of databases that have actually a bunch of small data. So talk to me a little bit more about data access and how you think about it, how and how people in this space think about it.
[00:11:21] Thomas Clozel: [00:11:21] I think exactly. So I think there is two sides of that access is.
[00:11:24] What type of data you want to access to. And the second one is hope. Do you want to access it to, so for the first type of data access, there is different types of data. The first one are what we call at Okin fit for AI that I said is that I said that I'm multimodal, longitudinal. Usually retrospective and high created by the best key opinion leaders in the world.
[00:11:43] Like data sets of 10 years of research on breast cancer. We single-cell CT scan, mammographic, everything and built with the mind of somebody that has questions in mind for us. This is very important and interesting because data scientists can just. Login and just trying to make models and understand things, research that sets the best in the world.
[00:12:03] Is there a big market for that? For the clients? Maybe not because it's really , but it's very rich in the idea. The second one is really like EHR data clinical features that are. That are mapped for a lot of hospitals in the world, and it can be used for pricing or market access for pharma, and therefore bring a lot of economic value when not only scientific value.
[00:12:22] And of course I bought for much larger prices. And so this is do type of different data. It's like very high quality data sets thousands of patients maximum, or just like big chunks of patients, EHR mapped in the world. And sometimes millions of patients. Of course, the second one is more big database and has a lot of economic value.
[00:12:41] The second way is how you access data. So is there is a, that economies that exist in the U S it's very clear. Hospitals are data brokers, they're vendors, sorry. They really sell that ivory day in the Europe with the GDPR regulation. It's not the same. And therefore you have a lot of hurdle to actually broke data.
[00:12:58] You also have a big hurdle to use the gas side technology around for the clouds that are storage. We have a big story now with the health data hub, it's a French. This institution that is trying to gather the best data sets of research of academies in France. And there was wanting to store that on Microsoft Azure cloud.
[00:13:17] And guess what? Big bro lambs? No way we are French. We not doing that. No, they have to take the data out, whatever, but this is another problem, but I think it's very, but it still reveals a lot of how you want to store that. I thought, I think there are we okay with the data brokerage and the economy in Europe.
[00:13:35] Not really. And I think we need to find alternative ways to access data without having to share them. And this is our idea with federated learning is we want to build a privacy preserving approach where physicians will trust you. Patients will trust you and where like people will exchange AI without sharing data.
[00:13:52] So the idea of integrated learning is you access that on premise that never leaves the hospitals. You can build an algorithm and the model on-premise within the hospital firewall. And then you can, the algorithm can travel and the model and being trained with other hospitals, but it completely respect the privacy of patients.
[00:14:09] And it doesn't include a rate identification risk. This is very important today. People say you took it to broke that out because we have anonymized data sets. So it's fine. The truth is it's impossible because too many people have a genomic genotyping that I said such as 23 and me. In the U S and control, and you can always cross it with the database.
[00:14:28] And therefore, whenever you have this library that have genomes, you cannot be perfectly
[00:14:33]Trond Undheim, Host: [00:14:33] Exactly. The moment you have genomic data, you can cross-match and you can at least know something about yeah. About the data. So data never leaves the premises. That's interesting. Before we go into to Oakland, because I do have a bunch of questions on what you are doing.
[00:14:48] Tell me a little bit about how you see hype versus reality, because. Clearly, this is a space where there's now accelerated investment, which means new actors are getting into the space that haven't really invested either in AI or medical. And now they're investing in AI for medical. So what does the space look like?
[00:15:08] Who do you see as the. The actors to reckon with. And are they only startups by the way, or is this also a topic where larger hospitals or larger medical and pharma firms really have a stake in the game at this point?
[00:15:27] Thomas Clozel: [00:15:27] It's a really good question. I think like first, when I'm sitting by the end, but I pharma industry starting to build off in terms of efforts of AI internally.
[00:15:36] And they really have a strategy of internal innovation, really about building huge teams, accessing data everywhere, regrouping their own datasets. You have to imagine that the pharma industry, so there's a big, hard time to regroup their own clinical trial data because they were working with Sierra. Was he on there?
[00:15:51] So they don't even have, but there's that X committee formed. So I think next phase is there is a lot of internal innovation. Pharma somehow are very ready. They have a lot of datasets. They have a lot of a big team. We call them pharma, AI, ready pharma, and some are less ready. But that's a, it's a really big thing around the space.
[00:16:07]Trond Undheim, Host: [00:16:07] Can you name drop? Is it
[00:16:10] Thomas Clozel: [00:16:10] Roche Novartis buyer? Probably the three, one top one and maybe J and J probably the fourth one that really has built. I have seen a strategy rush has seen the strategy of acquiring federal to access community clinics, EHR that are in the U S.
[00:16:24] The Hef from the show medicine was this like, genotyping technologies, and then they have patients in common between the I think it's around, I don't know, 50 cases to can, not sure, but the number, but that I have both Flatiron and foundation medicine and therefore they have a lot of capabilities to understand on the data side, so that access ready.
[00:16:42] And then they have. Hi, I'm Mark Lee, who is the head of PRC team who has a very light before dinner. Mika was somebody that knew he's a clinician too, like me and the methodologist and has a really strategy about how to build AI at scale. And he's bringing a lot of technologies inside. So I think Roche has been number one in.
[00:16:58] In terms of data access, and then we have Novartis and Novartis is building great stuff. So he's better on butts on the head of digital. That is really pushing efforts on the digital health, as well as AI side. It seems very important to us is that people remember it's very different and sometimes people mix up a little bit both, right?
[00:17:13] But digital arts is really something you can do with your mobile phone. AI is a really more broader space. But on the question of the IPE, I really think that today the question is during COVID how many drugs were built. Now you mentioned learning technologies really like at the end, I know that many.
[00:17:30]And when did we, the AI COVID as an example, it brought some triaged questions that were interesting, but however, if you're a physician today, it's very important to have to ask questions, but you know that if the patient is obese or have pulmonary problems or he's old, you already know you can already have a good triage by your own intelligence, or those can be, can really help.
[00:17:49] I find that
[00:17:49] Trond Undheim, Host: [00:17:49] fascinating actually, Thomas in some way COVID was extremely revealing on many sides of society and not just the medical science, but it certainly, it's almost like if only COVID had come five years later, I've heard, experts talk about the fact that synthetic biology, for instance, he's so close to its breakthrough moment.
[00:18:08] But we were almost, we were, we are five years too early. So the people who are in that space have had to patch up their work and refocus and kind of just scramble with the platform wasn't there. No one could just turn around and turn on CRISPR and tweak this button and then build, what the hype perhaps said that we
[00:18:27] Thomas Clozel: [00:18:27] could build.
[00:18:28] Exactly the same for drug discovery a little bit. So I think drug discovery has a lot of hype in AI and and the reality is there are great companies in silico, great company in Centro, good company, and other companies in regression, very good company to building new stuff, finding their own data sets.
[00:18:45] The problem first with drug discovery is they never really had the good. Training sites to train the models. If you want to be able to build the model to train your binding of a molecule to a, what do you need? You need access to the molecule data of the pharma industry. You need to the molecule pipeline and they never give this to you.
[00:19:02] And we always federated learning, find the technology to actually do that without sharing the data. But I think in drug discovery and drug discovery also has been too much black books. And I think you need to build that Quantic physics. A lot of things I was talking to who is the former CEO of of of benevolent and no, his he's a former VP of AI at Facebook.
[00:19:19] And he was like, probably we didn't. We didn't include enough like physics we, weren't just building, I go with them to predict things, but you need to, there is randomness, there is a lot of things when a molecule binds to a target and that's really it. So today is AI better than a really good chemist to build a new drug?
[00:19:35] I'm really not sure.
[00:19:38] Trond Undheim, Host: [00:19:38] Wow. Those are strong words from a startup founder in the space that has security venture funding. It's interesting. I,
[00:19:46] Thomas Clozel: [00:19:46] I think to say what I understand about this space. Yeah. I know. But
[00:19:49] Trond Undheim, Host: [00:19:49] no, that's why I like to, I hear from you, so let's move straight into, let's move into Okin for a while.
[00:19:56] Tell me just, why did you start it and what are you focused on?
[00:20:03] Thomas Clozel: [00:20:03] I were I started working when I was a physician, just because I wanted to bring you ways to build precision medicine. And the precision medicine is I was trying to find I was a lymphoma guy and I was trying to build predictors with genomic signatures and epigenomics and extras integrated to risk, to predict response to R-CHOP, which is the.
[00:20:20]The regular therapy, the classical reference therapy for the informa, but it was not working out that well. So that was it. I wake on L a was probably some now, and then I discovered there's always new technologies to try to make prediction, which is AI. So the idea for king was how can we make prediction that will help medical research?
[00:20:37]Then what we really realized is like, How hard it was for the pharma industry to work with academics and the best to access the right data sets and actually to be able to access the right models. And was also for some academies to have the right day capabilities respecting the patients that are, so the idea for king was really trying to connect everyone together.
[00:20:55] To really create unprecedented connections to build this new ecosystem where people can share AI models, making a very global AI that can actually generalize with respecting the patient's privacy. I talking when you work with us with that, we're pretty soon that are, it's not always true with other companies.
[00:21:10]And then trying to build this environment where there are scientists. Connect to physicians, physicians connect to other physicians with velvety learning and pharma connect to the hospitals to actually use the best AI that was built on site and improve how they develop that drug. So we really tried to focus on drug development and this is core system and gradually we understood what was the best use case to actually use all the insights and all that we were creating within this federated ecosystem.
[00:21:36] Trond Undheim, Host: [00:21:36] Fascinating. And what has the experience been, who are you working with and what do you find to be the best use cases for federated learning? At this point? I think we have
[00:21:46] Thomas Clozel: [00:21:46] a really flagship project. We have two factory product, but the first one is. Is really on the unprecedented level, it's it covers 70 billion of assets.
[00:21:55] It's called melody and middle D project is a project that is granted by a European fundings and actually regrouped 10 of the largest pharma in the world. Sharing models around drug discovery. Without sharing the data and using Okin technology. There is other like industrial partners, but okay.
[00:22:11] It's really the orchestrator on the federated learning side and the privacy and trust ability side. And we do this with our software called connect. This has been installed where people can just log in, have a remote access to data and actually launch a federated learning algorithm on this. I go within can be traceable.
[00:22:27]It can be federated and it's extremely secure. You never can know who puts which data on the model. And it's the first time. The pharma are collaborating on the molecular libraries. It's the biggest asset is a treasure that they're sitting on and never want to share that. And it's really breaking Seders, breaking copied, as you say, it was just to build something altogether and all the drama at the end will benefit from the same model.
[00:22:49] And just insights. This is so cool. Sky is the limit for federated learning. What about trying to to, to work on Alzheimer? This is everything fails. Every clinical trial spells pharma won't share the raw data, but why not building a federated learning algorithm that can learn about the insights of the real trials without taking the data out of the pharma prior wars, same for glioblastoma.
[00:23:11] No trial is positive and they are less brain tumors. Right? Why? Because we don't even, we don't even know if it's more shrinks on that on the city because it never really shrinks. So we need AI systems and we need to source insights in a collaborative way. Federated learning is open innovation and it's really about breaking silos everywhere.
[00:23:31] Trond Undheim, Host: [00:23:31] Thomas. This is fascinating. Tell me you speak about shared algorithm. That surprises me a little because I thought federated learning was mostly to share the data, but are they also sharing the algorithm? No. The real idea
[00:23:44] Thomas Clozel: [00:23:44] of federated learning is data stays on premise,
[00:23:48] Trond Undheim, Host: [00:23:48] but they're sharing something.
[00:23:50] And what they're sharing is the,
[00:23:53] Thomas Clozel: [00:23:53] or the data at the end, the federated learning, I go with them. They will benefit from it. As a model. Yes. Okay.
[00:23:59] Trond Undheim, Host: [00:23:59] But are they sharing the results of that algorithm crunching or are those so they are specific to each partner.
[00:24:08] Thomas Clozel: [00:24:08] The results can be trained every locally on each partners and in specific the resource of specific on there, but then the algorithm in on everyone and the performance.
[00:24:15] Do I agree with and can be trained and tests as it is by all the companies on new molecular assets? I'm with you,
[00:24:22] Trond Undheim, Host: [00:24:22] but just, for a lay person, the point is these 10 companies don't necessarily have to develop the same technologies all the way through. They can work on the same platform, they're sharing their data, but they can take away.
[00:24:36] So Roche could take away less than a and build product. A and Novartis could take away less than B and build product B and they don't necessarily have to collaborate, even though they have shared.
[00:24:51] Thomas Clozel: [00:24:51] Yeah. Yeah, exactly. And that's the thing is like the agronomist here in Russia is not participating, but in this case, like the pharma that are participating or shirts, some of the assets, but yeah, at the end, that is, I think the benefit of some things I learn of everyone assets.
[00:25:05] And the, and this is really where it's great. It's really like learning of different assets build on different settings. And yeah, this is really what it's about. And at the end it can be a shared model. So it can be a model that you can use for new molecules that you want to predict binding for.
[00:25:20] Oh, you can use for for Alzheimer. It would be insights, new biomarkers that could be shared to stratify patients for future trials. Hey, we discovered that this part of the brain credit response to treatments, and we build this with all of you. So you can use this pretty is a little quantitative biomarker.
[00:25:36] To stratify your patients in your next trial. This is share knowledge at the end or shared insights or shared model of prediction. But I think it, it never uncovers the, what is really priceless for the pharma, which is like the raw data.
[00:25:50] Trond Undheim, Host: [00:25:50] So tell me a little bit about what the results have been so far.
[00:25:53]For you it's early days, for but for others. So give me some specifics about other AI. Projects in medicine. And what kinds of early results have we seen? So we just talked about, COVID not very impressive, but for some other things, I just give you
[00:26:08] Thomas Clozel: [00:26:08] the example of something we built at origin, because it's when I know best mesothelioma, mesothelioma is a cancer that people die within six to eight months in median.
[00:26:18] It's, the part of the Lank that is usually linked is that the cover, the lungs, and it was linked to our best tools is when people were going in the minds. And the skin story is also linked to a mutations, could by 21, which actually makes the incidence not decreasing that much.
[00:26:30] It's a very bad cancer and no one knew how to treat it. For many reasons, not the right chemotherapy, doesn't go really well into the tissue. And we actually had access to the largest cohort of patients in the world, thanks to our federated learning technology and the trust we create. We had 3000 patients and what we had is.
[00:26:46] The biopsy baseline and the response to treatments and the outcome, the survival. And we say, let's try something hard. Let's try to see if we can predict the survival using our machine learning platform and the technology we develop in pathological shoulder, which pre processes, the images in very little dials before applying a CNN neural network.
[00:27:06] And we are literally like, say, yeah, can we predict survival using just the biopsy baseline before treatments? So when you think, when you give this to a pathologist and he can think the tumor, when exactly he looks at his microscope, he cannot really told you if he's the patient might survive six to eight months or eight to 10 or 10 to 12.
[00:27:21]And no idea he can see if there is some signs of infiltration or malignancies or division of the cells, but he cannot do that, but I work our model and there were algorithms that we could miss and it could. And what was very interesting was not that we had actually the possibility to predict survival or using baseline pathology images, putting the response to treatment as the treatment is homogeneous.
[00:27:41] What we had here is we could really understand how we could do that. What was interesting for us is to talk to the QPR leaders and show them the little tiles in the image, helpful to make the prediction happen. And we discovered that there was a pattern and the pattern was. The tumor micro environment, the periphery of the cells, what is around the tumor is the most important part to build the model.
[00:28:04] And we confirmed this with the best Cuban needles. And then we did, we discovered some cells, pleomorphic cells that were really important to, to make this prediction happen. And we went. Deep dive into biology, trying to concrete it. So we discovered that this trauma of the cells is important for survival and can determine new subgroups that were not known before.
[00:28:22] So we can determine with a high, new, high value subgroups that can be used to reclassify the disease in a clinical trial in general as well. And this is so exciting and we are, you can go to new targets, Google go further and where you actually make a real medical breakthrough using a prediction made by AI and collaborating with the best peers in the field.
[00:28:41] Trond Undheim, Host: [00:28:41] That's fascinating, Thomas. I'm really happy about that. Tell me a little bit about how you how you see the market evolving, because we have talked vaguely about the, my sense, and I don't have the data in front of me, but that the investment levels have gone up in this space. How do you.
[00:28:58]And do you look at that at all? Is it relevant to you? To what extent this market for AI in medicine is growing at what percentage? Like how do you see this market evolving more important?
[00:29:09] Thomas Clozel: [00:29:09] It's a very good question. I think there's two sides of here. There's a side of the AI market in healthcare and then the data market, it's always a little bit intrigued in. So the, that time market exists, it's a buying data. For medicine everywhere for payers, as well as pharma is a big thing. Most of the lag that a clinical data maps, pricing and market reasons, a lot of value here, a big business acquisition of Flatiron was a great company happened, but there is concert AI, a lot of new company like that.
[00:29:38] They also do the same, a lot of Trinity's with company here. When you also do that, you really, you also on the. That the clinical trial operations. Can I work with patients based on this data for my clinical trials? And that is something very important too. It's one of the priority of very ly building of a registry to actually be able to do that.
[00:29:56] And really like the baseline study is very, whenever you touch to pricing or clinical operations, you hear your. Big money. And the market is mature, right? You have teams in place. You have Birgit in place lines. There is a line in the budget is buying real world evidence, data, buying, pricing, market access data.
[00:30:13] That's okay. When you go to AI, I think it's a really new market and and there is no really lines of budget here to do that. And of course, if you build a new molecules and if you succeed. You have big line of budget that will be developed. But a lot of things today in AI is a repurposing drugs, which is always completed on the IP and patent side.
[00:30:30]It's not easy to defend these patents. And I think like whenever you touch two more like different R and D of the pharma, I think of course the budget, even if you're super innovative, the budget are less important. And therefore the market of AI for drug development, for example, I think is gonna really blow and grow.
[00:30:46] It's already, it's going to grow in the blow. But it's, it will take a few years because today the farmer has to open to these technologies to really make it. But the reality is you can really change how you understand. I think of trials with AI and you can do a lot of things, but I think the markets would be more mature when these tools will be ready to get to, we get out to regret it.
[00:31:03]People who wants to stamp of the FDA or the MEA, and also I think, yeah, it's going to grow. I think the budget will change and have this. But today, I mean like the market is very segmented. It's really pricing and operations and R and D on the other side. And depending where you fit you possibility to grow on the scale of revenues is not the same.
[00:31:22] Trond Undheim, Host: [00:31:22] So you, you were hinting at this, that two things, one big pharma isn't necessarily in the best position right now to handle this. How are you thinking that? There's a bit of a fear or even like wishful thinking that this is going to take much longer to come to fruition and that the big pharma kind of playing the game that the automotive industry was playing with electric vehicles.
[00:31:48] They're saying deep down, we know this is going to happen, but it's not really in our interest to have it happen too soon. So we're not going to turn our entire business model around, throw out everything we ever knew about clinical trials and go this digital route. Because what if it takes five, 10 years then you know, all of this revenue that we could have had, is that the game they're playing or is it a more fundamental lack of understanding or are there actually not playing?
[00:32:14] They're just more realists.
[00:32:17] Thomas Clozel: [00:32:17] I think they're all very different than that. But I think just to take you on that and energy of of automatic cars, it's very interesting how the harder the perception of AI for automatic jazz in pharma for, but it's completely different. For example, when you drive your Tesla, are you asking.
[00:32:30] Hey please test. I give me the AUC accuracy. Give me all the model facts of your autopilot drive. I want to be sure. And then, oh my God, 0.7 87. I'm not going to drive, you don't actually ask the same stuff. When you go to a pharma and you build the AI, you want to send them a little facts, which patients were trained on how you generalize it.
[00:32:48] What's the power of it. First I think the requirements of the pharma is very different, but I think the game of, so the pharma I think is. Starting to understand how to play with the eye around. I think the pharma is there is not much acquisition M and a space in this space yet. So I think he's just want to try out different solutions around the other lot of things already frauds, but I think the problem is pharma and the day to day is a control problem.
[00:33:08] It's very different to work in the pharma when you have Google, it's way more cooperates. And I think today, I'm not sure the pharma is attracting the best talent in AI. If you're a data scientist and you're both number one on , you probably want to go to Google. And that's where a company today. And
[00:33:25] Trond Undheim, Host: [00:33:25] there are those that say that.
[00:33:28] It's just a question of time and actually trategy before big tech will take over pharma and not the
[00:33:34] Thomas Clozel: [00:33:34] other way around. In a way, yes. If you see Google will be able to make clinical trials with 20,000 patients. And I think me, I still have, I really love pharma because the thing is they're the most innovative company on their way.
[00:33:44] And I think actually really great as a scientist are starting to grow. And I think in two years, three years, probably the best at the census want to go to pharma and make sense of it. But I think today there is still as place for startup because system where there are really between the tech and the pharma, this is us we're right between both.
[00:33:59] We take both of both worlds and we are neutral, but we had this cultural AI fit very geeky. And I think this is for the farmer. I think they have all interests today of not internalizing these efforts, but trying to keep things around them. And and play around with, I think the strategy of the farmer today is they know it's going to be super attractive to the best talents in two to three years that they're not putting huge budget because they want to wait and see, which is actually makes sense, right?
[00:34:22] Because a lot of things that is very over-hyped and doesn't really fit and and maybe I think the two to three years and may made some of the acquisition on the AI side, but I would not expect it before. Nice.
[00:34:33] Trond Undheim, Host: [00:34:33] Fantastic. So Thomas, how does this then apply to governments? You are yourself involved in an EU project, Eve finances, Steve finances, and plethora of projects, both.
[00:34:43] Yeah. On AI for health. And then clearly also has very strong views on privacy. And you mentioned early GDPR, but there's certainly a bunch of other health regulations that apply in the EU. And then of course, in the us with HEPA and. With HIPAA and other regulations. W what is government, if you were advising governments right now, what, if anything, can they do apart from watch this space unfold at this point,
[00:35:10] Thomas Clozel: [00:35:10] they can take, I think they can take government decisions.
[00:35:12]So I think the example of France. Very good physicians public data sets the premise who does the data sets be done to when the data, if you do a CT scan in Paris in the public hospitals, that's not happening in Paris, but that happened to real life. Are you going to do that? Does it belong to you the CT scan or does it belong to the country because you paid zero for it, right?
[00:35:31] Or you pay some taxes? I think no one knows. Is there a lot of confusion about who that belongs to in Europe in many different levels, even in the U S it's not that clear, therefore I think as a government, we could take the decision saying every data produce in the public hospitals belongs to the government.
[00:35:47] You can sit on the opt-out in some ways. But you'd be done to the government and you building a very fundamental assets about like having regroup, mapped, understood all your house that are built in your social ecosystem and why not. But you need to make it clear. It means that it belongs to the hospitals and the government does not belong to you.
[00:36:05] You cannot tell you that are online. Because you can't do this today. And I think that maybe it's not a very liberal choice, I don't know, but this is a choice that could be taken on a high level by government and is enough confusion about who owns the, what. To do that. There's confusion about
[00:36:17] Trond Undheim, Host: [00:36:17] who owns what, but there must also be some confusion in governments about, do we know enough to regulate this right now?
[00:36:24] Like I'm sure they're scared of messing things up, both for their established powerhouses, there France, for instance, and Switzerland and other countries that do have big pharma, I'm sure they don't want to. Do something that could become a big problem and vice versa, they don't want to get the population against them.
[00:36:43]If they say, Hey, you're right. And there, privacy laws in the EU, so this is it's a little, it would be, I
[00:36:49] Thomas Clozel: [00:36:49] if he would react well in France, I could be like yeah, there would be some strides for sure, because. With French do. But and in general, yeah, I probably to thinking of that out is a provision of Liberty, but in France, whenever I think it's something that you would give for the goo we're good.
[00:37:05]It's better research is they could help you or your operations and the hospitals to a better yeah, but of course, like the thing about having data that are not your own is the payers paradigm. Everybody's afraid of payers set primes and taxis on that. So the payers would weigh payers will pay.
[00:37:20] And of course, some things that everybody has in mind.
[00:37:24] Trond Undheim, Host: [00:37:24] Thomas, can I get some prediction side of you? If you look at the next decade in your space, AI for medicine what's going to happen? What, how fast is this going to move? Considering all the things we've talked about, the technology opportunities on one hand, the government constraints on the other, the innovation in the startup space, the existing pharma industry, which is slightly entrenched, but maybe, also waiting for the right moment to either start acquiring and understanding more, or obviously to react.
[00:37:52] If big tech starts to make moves into pharma, then they would have to look more like big tech. But how fast is this going to unfold? And with what consequences for changing that. The laws of fundamental science and benefiting, sick patients, which I think is both of our concerns.
[00:38:09]And certainly, should be a very big concern. COVID is one thing, but there are so many diseases where like you pointed out in various types of cancer. You could see it, the glass half empty view would be, we haven't made that much progress in cancer in 30 years.
[00:38:26]Thomas Clozel: [00:38:26] I really believe like AI.
[00:38:27]It's really good question. It's really broad. I believe like first day is in the full spectrum. AI will accelerate many processes in hospitals will accelerate operations that will benefit to the patients directly. We'll probably be better in some diagnoses that physicians that are hearts will better detect.
[00:38:42]Patients for rare diseases, patients today, sometimes they never did it for rare diseases that will be predicted on the CT scan a spectrum of rare disease. And you will have diagnosis rather than this is something we'd never think about. The research federated without competitive silos, people sharing.
[00:38:56] I go with him since to Hakim technology and federated learning pharma, we'll use some insights here and there and some technologies here and there. You won't be fully, completely integrated in the next five years work pharma workflow. I don't think so. It takes 10 years. We'll take probably the quantum computers to accelerate everything.
[00:39:10] Suze. COVID on computer is a very important step. But I think pharma will have something nerdy here and there are some insights used and we'll use AI approach to access that as well. Probably in the privacy preserving where as we believe I think like patients will have, we own that data and do some blockchain technologies, not really AI, but you can be doing to sell that data and make money from it.
[00:39:29] I think a lot of patients will make a lot of money from their own other set because the data sets will become a bigger and bigger commodity. And people will give a lot of price and money to own that. And yeah, I and find it yes. And do the payers will, a lot of companies will, we'll both play and with the payers and pharma game, which is a complicated game to me, and and the payers, the spectrum between the payers and whatever they do is your data is going to be more and more complex because what is the interesting prediction, if your payer is who is going to die?
[00:39:56] Very early, we start going to the hospitals. It's pretty, only pretty fancy. I was very interested in and yeah,
[00:40:04]Trond Undheim, Host: [00:40:04] It's it's a fascinating time we're living in because presumably this decade will really. Whether it goes as fast or slow, as you said, or it goes much faster, like hype says or are far slower our straw man, pharma, executive hopes that you know, that it'll take a little longer, how.
[00:40:26] Do you make these determinations? I always ask my guests, how do you on your own track, these trends, how do you stay up to date? How do you glean those insights that are needed to make the predictions you just made? Can you give. The listeners some idea of in this field. Do you just track other startup founders?
[00:40:46] Do
[00:40:46] Thomas Clozel: [00:40:46] you, yeah. I For me to meter into mixer for the tracking or the companies around having an eye for collaborative efforts, AI is really about collaboration. The CEO of in silico at Zachariah, somebody that's really trained to create the connection and the links between every farmer in the world, every company.
[00:41:00] So we have ecosystem called in the U S to speak on the regulators with one voice. And we wrecked Russia and we is a numerate well in silico. And really this guy has been central into linkings this air force and having people discussing around the table. So I think we all know each other and we are quite collaborative.
[00:41:17] I don't think that there is no secrets. We have the, we are very open source minds, people at Agnos, like share, share what you succeed in and share your, what I had. I had a brunch, I had the brunch this morning with Adam from . Hey, man, what's working with you. It's hard. It's a complicated business. Anyway, so anyway, so at first I just got things very openly.
[00:41:37] I think then trying to track what's the thinking change in the pharma industry too, because pharma is going to be the driver to build new drugs and they're doing amazing stuff today, but really understanding how they think about AI and having discussion at different levels. From the very top levels, he levers to two people that are the data scientists within this company is very important sides to take.
[00:41:54]And and I think like the last thing is about, yeah. Understanding what's the impact on the peer review papers, we have publications, nature medicine, and the new papers that go out. And sometimes you have great discoveries that don't generalize. And I think like a lot of, no to be question is if you show something, is it robust?
[00:42:09] Can you use it everywhere in the world. And we should say my curiosity in the same agency. So really with the same performances. And when you say show me usage, so everything, and I think it's this calm sense? There is more, sorry, there will be a lot more payers will be ready to pay.
[00:42:23]And that will drive a lot of things because today, like the fact that payers don't pay back the diagnosis AI made by AI it's really gives a lot of it's a big, it's a big problem for people. Dignity's company in AI in pairs on pay. The business is really hard.
[00:42:39] Trond Undheim, Host: [00:42:39] Yeah. So the business model, right?
[00:42:41]That's one aspect we had, didn't spend so much time on, but maybe I can come back to you later on. We'll talk more about the different emerging business models within this space. And maybe I could just ask you in one minute to explain what you think is first off, maybe the easiest what's Alcon's business model, where do you.
[00:42:59] Earn the money because where the money's coming from is really gonna determine the interest of big pharma as well. Because if it's a business model that fits with theirs, that's one thing. If it's completely perpendicular. Now they're going to fight against it. So how do you see those business models evolving?
[00:43:19] Thomas Clozel: [00:43:19] I think we've been lucky with Okin because we kept we, we have really good revenue, track revenues and traction. The idea is we kept quite a laugh spectrum of products because you need to adapt to pharma that are already. And the one that I know that I already do, you actually want to be a critic.
[00:43:30] So I think the first step is really being a critical friend to every farmer, whatever stage they are. And and today our business model is really different levels. It's a data platform. He's actually seeing models of prediction and he's actually using a Oki knowledge to use these models within a clinical development workflow, into clinical trials or into patients notification for conversation.
[00:43:50] And so we have given three levers and the three kind of fits at different pharma biotechs. Stages, of course the tide's never brokage it's remote access and execution of models by pharma to the scientist. So I think that I could do the fact that we build this Relayr us really helped us out.
[00:44:03]And and and we have, we are very fair and transparent on the results and where we expect and nursing the biology, and we go in deeply. So that kind of pretty worked out and seems to be a really scalable business model is three layers. I think it's very hard. It's called. approach having technology that is multimodal multi disease and that's really super focusing.
[00:44:24] And I think this is something that is a choice, buy, sell revision, as you deny we seem far, but sometimes it's hard because when you raise money, but it wants you to be hyper-focus on something, right? So it's a truth. Do you want to be super vertical or are you okay to be quite agnostic and a reason?
[00:44:40] It's a really important question in the business of AI startup.
[00:44:44] Trond Undheim, Host: [00:44:44] That's a very profound, and I think that is going to be a big question that venture investors will be asking a bit of pharma will also be asking corporate investors will be asking themselves that question because, one thing that I actually heard.
[00:44:58]In the MIT circles, working with pharma was, there were actually some of our big pharma companies coming to campus and they were advising startups to not listen to the seas in this case, because the typical VC angle on things is closed down, focused on one thing, do that well, and then, yeah. Get your company, a good price in some, as some good money and then just do that, get that thing into the clinic, and then we can start talking,
[00:45:23] Thomas Clozel: [00:45:23] but we had to have different.
[00:45:25] Yeah. We had the chance personally to venture be a venture capitalists that understood that they want you to also explore the business with us. And they understood the company of being a hypothesis driven. When I raised money, was he going for Google venture or women investors? I went to San Francisco with GL and we told them we are testing this business model.
[00:45:41] Is this the right one? We are not sure. And we, I don't know. And and I think we've been it was quite refreshing to hear for them. And I think, yeah, just no one knows exactly what is the future business model of AI was the right one. And I think we have to tell you as it is and not oversell, French, we never oversell.
[00:45:56] It was something we should maybe learn to do. No, I,
[00:45:59] Trond Undheim, Host: [00:45:59] in fact, I would argue you shouldn't learn because you know what the, what these farmers were telling me in turn and, honestly, they are partly to blame. So I don't know exactly why they blame the VCs, but they were saying once we get to the company and the presumption was, we don't invest early enough.
[00:46:15] The company is not as interesting as it was five years ago. And they were complaining that the VCs were destroying their companies, making them less platform based and much more focused on like single disease or single track mind, which in the end was, is an impossible decision to make that early in, in that kind of company.
[00:46:32] Yeah. Anyway.
[00:46:35] Thomas Clozel: [00:46:35] So the way to put it is like AA format always need to run and reinvent themselves really hard. You need to run to my cash and to you, if you have no cash, you need to grow. But today, like the thing with with AI is revenues cannot be the only metrics. It's not a site's business to, to scale your company and to raise.
[00:46:50] It's a lot of different stuff. So how you actually like the data sets access. You edge technology. And there's a lot of things that being taken into account. We had very smart VCs that could understand that too. But of course revenue is still like the best proof is how strong are you on the market?
[00:47:05] And I think it was still like, people shouldn't say revenues as important. I'm going to have a really great director if you ever need that access to make money. And you need to think about how you can scale your business and
[00:47:15] Trond Undheim, Host: [00:47:15] yeah, it's not either, or look, Thomas. It's been fascinating. Thank you so much.
[00:47:19] Thank you so much for your time. No problem. I love, yeah. I love the conversation. I hope to have you back on, we're going to track on features. We're going to be tracking these field very closely. So I hope to do have many of your peers in the time to come.
[00:47:35] Thomas Clozel: [00:47:35] Thank you so much. Have a really good day.
[00:47:37] Cheers.
[00:47:39] Trond Undheim, Host: [00:47:39] I just listened to episode 55 or the future-wise podcast with hosts through an honor and hype, futurist and author. The topic was AI for medicine. Our guest was Thomas Clozel, the CEO and founder of Oak and the federated data network startup with boosting AI for medicine. And this conversation, we talk about integrating AI and system biology to enable breakthrough medical.
[00:48:05] Moments we explore how machine learning can be used to prove medical and biological research in Ocwens federated learning approach. The data stays with the owners, but the learning models trap we discussed. The data heterogeneity in healthcare, the need for interoperability and we touch on hype versus reality.
[00:48:29] My takeaway is that AI for medicine is here to stay. It has come far, but has bumped into some fundamental obstacles, interoperability. Explainability privacy and transparency, but that need to be resolved before reaching its full potential. That's even more important than evolving the base technology to become more efficient.
[00:48:54] This is why federated learning is such a crucial experiment. Can it work? Will it satisfy everyone? Time will show AI is not monolithic and neither is medicine. There seems to be many contenders for glory, and there are many puzzles to solve. They won't all go away this decade. Thanks for listening. If you liked the show, subscribe@futurize.co or in your preferred podcast player and rate us with five stars, futurizing preparing you to deal with disruption.
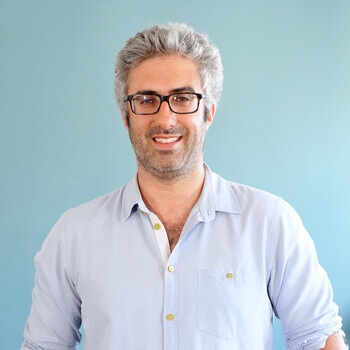
Thomas Clozel
Founder, Owkin
Biography. Thomas Clozel is a former assistant professor of oncology/hematology in Paris, and had co lead different projects focused of prediction of resistance to chemotherapy and ways to reverse it at Weill Cornell Medical College.